
Author
Peter Iansek
CEO & Co-Founder
Table of Contents:
- The Flawed Foundations Of Customer Understanding == The Flawed Foundations Of Customer Understanding
- The Curse of Knowledge == The Curse Of Knowledge
- What This Has to Do with Chatbots == What This Has to Do with Chatbots
- The IVR: A Legacy System That Mirrors Business, Not the Customer == The IVR: A Legacy Systems That Mirrors Business, Not the Customer
- What the Data Reveals == What the Data Reveals
- Unlocking Customer Reality == Unlocking Customer Reality
- The Bottom Line == The Bottom Line
A friend recently messaged me about a particularly frustrating experience he had with a customer service chatbot and asked, very sincerely, “Why do these things still suck?”
From the screenshots he sent, the experience was truly bad—the bot misunderstood him completely and was laden with rapport-building statements that only made things worse.
The thing is, he’s far from alone.
The Wall Street Journal recently reported that customer experience ratings are at their lowest point in the last decade, citing “fallible customer service chatbots” as a key driver. At the same time, within the CX industry, AI is being promoted as the solution. But according to Harvard Business Review, most AI projects fail—at a rate of up to 80%, more than double the failure rate of corporate IT projects a decade ago.
Over the past ten years, organizations have dramatically increased investments in customer service and customer experience (CX), recognizing their importance in driving business growth and loyalty. So why aren't things getting better?
Having personally spent over a decade operating, building, and implementing technologies for contact centers, I’ve experienced firsthand that there are a number of contributing factors. But one stands out as the most foundational:
Most businesses don’t really understand what their customers are asking for.
Put another way: the “human-grade” understanding businesses rely on—often rooted in experience, not data—just doesn’t cut it anymore, especially when it comes to powering experiences through automated technology.
That might sound harsh but when you examine how contact centers understand and measure what customers want, the issues become clearer.
Let’s break this down further.
The Flawed Foundations of Customer Understanding
To understand why so many automated systems fail, we first need to examine how businesses attempt to capture and understand customer needs today—and where those efforts fall short.
Foundationally, the contact center exists to service customer needs, so how are needs measured and understood?
For the majority of contact centers, especially at the enterprise level, understanding what customers want and need is based on the reason why they’re reaching out. Customer demand is the single biggest driver of cost and customer experience in a contact center, so it makes sense to start here, especially if you’re looking at how to automate specific customer requests.
From a measurement standpoint, there are several approaches typically used.
Operational Reporting provides the business with objective statistics into customer demand volumes—for example, how many calls were received yesterday, how many were answered, how many hung up, and how long customers waited. These metrics are reported numerically (e.g., 3,128 calls answered, 238 abandoned), but they don’t explain what was driving that volume—why customers were reaching out in the first place.
Dispositions / Wrap-Up Codes are applied at the end of a service interaction, when a frontline agent typically has about five seconds to select a reason for contact from a pre-defined dropdown list. This list is often built from internal knowledge and categorization. The dependency here is twofold: that the appropriate code exists, and that the agent has the knowledge, recall, and time to accurately choose it. While this is a step toward measuring intent, it’s still interpretive, manual, and doesn’t scale. Having spent many years as a contact center analyst analyzing dispositions, the most common issues are: (1) the agent selects the wrong reason for contact, and (2) the agent selects a broad category i.e. Billing, which offers no context or insight.
Customer Surveys are another widely used method. In the contact center context, the most common example is a “post-interaction” survey asking customers to rate their experience. While surveys can provide objective, customer-centric feedback, they don’t capture the reason for the contact itself—only the sentiment about how it was handled. Survey response rates are also notoriously low (often <10%), and when offered selectively, results can skew toward only the happiest or angriest customers.
Speech & Text Analytics tools mine customer interaction transcripts for behavioral insights. These systems appear promising but in practice run into limitations. Queries are often keyword-based: a search for Payments may yield thousands of hits, but offers no context—is the customer trying to make a payment, change a method, fix an error, or confirm a refund? Just like wrap-up codes, these systems bucket language into vague groupings that don’t mirror how customers actually speak. They also can’t reliably connect utterances to distinct interactions, so it’s difficult to quantify how many calls were about something in any specific, reportable way.
IVR Reporting captures how many interactions flow through each option in the phone tree and is often used as a proxy for intent. But this assumes the options match customer reasons for contact—which, in reality, they rarely do. The insight provided is only marginally better than wrap-up codes: “X% of customers pressed 3 for ‘account help’” still doesn’t tell you what they needed. And like the other methods, it reduces complex customer needs into simplified internal buckets.
As you can see across all of these methods, there are unique challenges in measuring and understanding why customers reach out. But there’s a deeper, more systemic issue—one that undermines even the best reporting setup.
The Curse of Knowledge
No matter how optimized these systems become, they’re all affected by a core flaw: businesses interpret customer needs through the lens of the work they do for customers.
We call this the curse of knowledge.
If you ask a Contact Center SME, “How well do you know why customers contact us?” they’ll likely answer confidently: “Like the back of my hand.”
And they're not wrong-at least from their point of view. Many contact center leaders have spent years on the frontline, starting as agents, progressing through the ranks. They’ve resolved thousands upon thousands of customer issues firsthand and built a deep understanding of the business and how it operates.
This internal knowledge is not up for debate or criticism. It’s valuable.
But here’s the catch:
That knowledge is centered around what the business does for customers, not how customers articulate their needs.
It’s a subtle but critical distinction.
I remember earlier in my career working in contact centers, being asked to list out the reasons customers contacted support to inform project prioritization. The list looked something like this:
- Membership Updates
- Payments
- Hospital Admissions
- Review Coverage
- Claims Inquiries
Exercise: ask a Contact Center SME in your business to do the same thing - I’m highly confident that the list will look similar in terms of categorization.
The reason for this is that they’re familiar categories that reflect the work the contact center performs. These are the internal business labels but they don’t reflect the language customers actually use when they reach out.
This is the “curse of knowledge” in action. It’s when internal expertise becomes so ingrained that it prevents you from seeing things through the customer’s lens.
A good way to test for this is to flip the exercise:
Exercise: ask your SME to list out the universe of customer requests, exactly as customers would articulate them, and to aggregate those by frequency.
You’ll quickly run into two major challenges:
- Mental model shift – It’s hard to unlearn the way you’ve always framed the work and instead see it through raw customer language.
- Cognitive limits – Humans simply don’t have the capacity to process, interpret, and store conversation-level insights at scale
What This Has to Do with Chatbots
This misalignment in language and understanding is the silent killer of chatbot performance.
Chatbots can only do what they’ve been trained to do—and they’re being trained by the curse of knowledge.
For example, a customer doesn’t contact support and say Payment Inquiry (how the business categorizes the work).
They would explain the reason in their own words, based on their experience and context, for example:
“I’m trying to update my payment information online and it’s not working”.
Now let’s look at what a high performing agent would do to manage that interaction.
They’d ask effective questions to understand the issue:
“Did you receive an error message?”
“What payment method are you trying to use?”
“What is your payment frequency?”
They’d diagnose the issue:
“To pay via bank account, you need to switch to annual billing, first.”
And they’d guide the resolution:
“Would you like me to walk you through it?”
For that unique customer stated inquiry, there is a distinct triage and resolution pathway to provide an outcome for the customer.
Without this granular, customer specific context, a chatbot is essentially guessing as to what the issue is and responding based on its training, which doesn’t have this specificity.
This is why the common experience for consumers is that the chatbot doesn’t really understand what you’re asking and provides responses that may generally apply to your circumstances (i.e. Payments) but not to your specific context.
The nuance here is really important, if we look again at the category of Payments customers will describe this in a myriad of ways all of which require a unique resolution pathway. Specifically an Issue with a payment, Ongoing issue with a payment, Question about a payment, Request to make a payment, Change a payment method etc are all unique and would require a different set of steps to help the customer achieve an outcome.
Lacking this nuance and specificity is why the experiences break down.
The IVR: A Legacy System That Mirrors Business, Not the Customer
Another long-standing example of this disconnect is the IVR—the dreaded phone tree customers navigate when trying to complete a task or reach a human.
Most people don’t need to think too hard to recall a painful IVR experience. Pressing “0” repeatedly or saying “agent” is practically muscle memory at this point.
Why does this happen?
Because the phone tree is a mirror of the business’s understanding of why customers contact. It’s designed to route you based on internal understanding and “work” categories, not what the customer is actually trying to do.
And those categories—“Sales,” “Service,” “Account Support”—are often misaligned with how people actually think, talk, or what they’re trying to achieve. The result? Wrong departments, repeat calls, dropped resolutions—and frustration.
The same misalignment in language, understanding, and structure that hampers chatbot experiences is baked into traditional IVRs.
You may be asking yourself at this point, “how much of a gap is there between business understanding and what customers actually want?” and the short answer is, much more than you think.
What the Data Reveals
We recently implemented Operative Intelligence with a leading financial services organization and trained a model to analyze interactions for just one functional area within their customer service business.
The models are trained to objectively identify and classify the universe of ways in which customers articulate their requests. The models analyze and categorize entire phrases of customer speech, not keywords or utterances.
The result: 457 unique customer-stated inquiries were identified for one functional area of support.
Each one was a distinct, fully structured reason why someone reached out—expressed in their own words, requiring its own pathway to resolution.
Other customer examples include:
- A global FinTech company has 986 unique customer inquiries
- A multi-brand payer has over 2,000 unique customer inquiries
This isn’t the exception—it’s the norm. And when you look at this level of nuance, it becomes obvious why human recall and internal assumptions can’t scale.
Further, why there is such a gap between what automated systems are programmed to do and what they can actually understand. For comparison, a contact center might "reliably" disposition 30 - 50 unique reasons why customers are contacting support vs the hundreds that are actually occuring.
Most organizations have never seen this level of granularity before. Because they haven’t had the tooling or methodology to uncover it.
The data doesn’t lie: the customer understanding gap is wide, and automation built on assumptions can’t close it.
Unlocking Customer Reality
The perspectives shared throughout this piece are not theoretical - it’s grounded in direct experience of working in contact centers and facing these problems.
This foundational problem is why we built Operative Intelligence and the idea for it began in a contact center over a decade ago.
Back then, my co-founder and I were working for a large payer and despite the mountains of data available to us in the contact center, alongside what was then leading technology, there was no reliable way to know why customers were calling that business. To the extent that if you asked 5 different people across the business why customers called, you got 5 different answers.
This understanding (or lack thereof) was driving business prioritization and decision making.
Out of necessity, our co-founder James developed a methodology to surface the real reasons for contact.
This process involved having frontline agents listen to calls and manually transcribe the customers' stated inquiry, verbatim (this was long before ASR was readily available or feasible for the contact center). The customer stated inquiries were transposed onto post it notes and then plastered across the walls of a conference room (we’ve included an image of what this process looked like below).
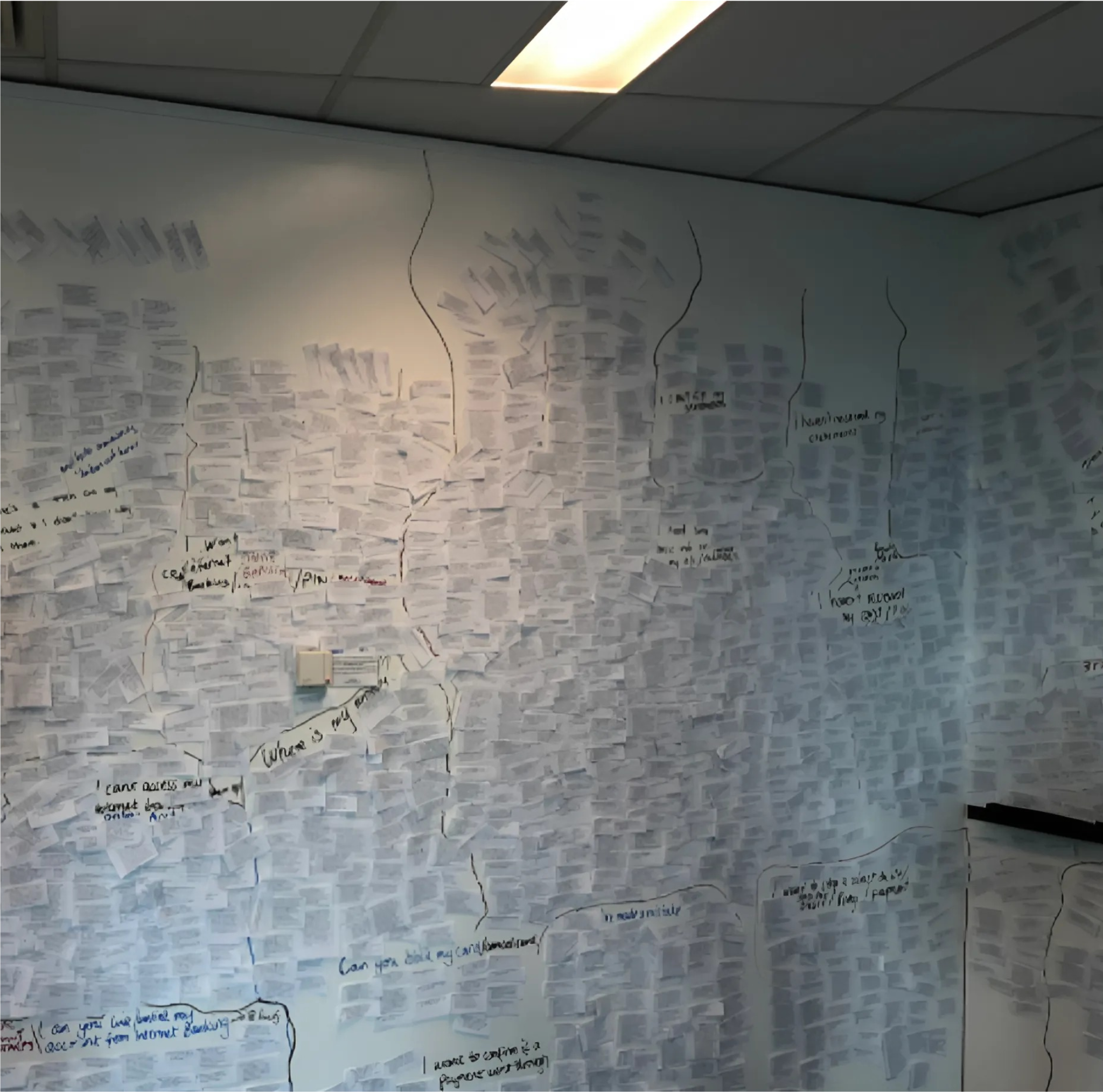
Customer verbatim being classified into unique customer requests
A team then spent one month locked in the conference room, going note by note, looking at how customers articulated their requests, in their own words to create inquiry groupings. Not with business labels but with actual customer language.
The team then applied the 5 Whys technique to uncover the root causes behind each customer inquiry—what really drove the contact in the first place.
The process was manual, painful and time-consuming but the outputs delivered the first view of customer reality for that business, driving a multi-year transformation that increased NPS by 5x.
The breakthrough was being able to objectively demonstrate to the business what customers really want, in their own words and using this to put the customer at the center of business decision making, without any ambiguity or guess work.
Given the outcomes that were achieved, the methodology was refined and improved in contact centers over the subsequent decade and in 2020 we founded Operative Intelligence to automate and help scale these outcomes for contact centers globally.
Fast forward to today, this same process that historically would have taken thousands of hours of specialized human effort and months of time, is now being powered automatically for our customers.
The Bottom Line
There’s a simple truth that underpins everything discussed in this piece:
You can’t automate what you don’t understand.
And even more fundamentally—you can’t automate what you can’t reliably measure.
Most chatbot and CX automation failures stem from this single point of failure. Businesses are attempting to automate against categories and assumptions, not actual customer-stated needs. Without a clear, structured understanding of what customers are really asking for—and how often—solutions are being designed in the dark.
It’s not that AI doesn’t work.
It’s that we’re feeding it an incomplete—and often incorrect—view of customer reality.
Until that changes, the experience won’t either.
This is the gap we’re solving at Operative Intelligence—giving businesses a way to see what their customers actually want, at scale, and use it to design better service from the ground up.
The future of automation isn’t about just adding more AI.
It’s about giving AI the right fuel: structured, objective customer reality.