![Call Center Analytics to Know to Improve the Customer Experience [2023]](https://assets-global.website-files.com/6152b238a476cfcf87fcf022/653f80bad113b66f102b15d7_63b5867fd1a76a0c05221257_call%2520center%2520agent.png)
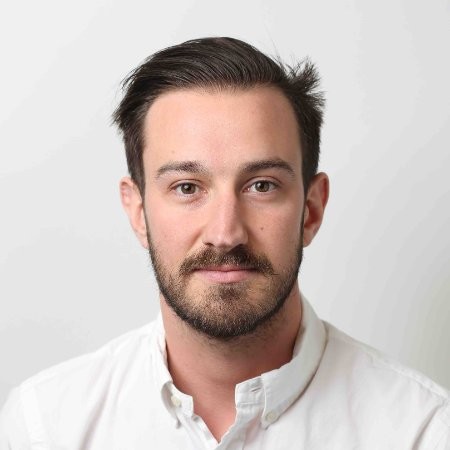
Author
Peter Iansek
CEO & Co-Founder
Table of Contents:
- What is Call Center Analytics? == What is Call Center Analytics?
- Call Center Analytics Benefits == Call Center Analytics Benefits
- Types of Call Center Analytics == Types of Call Center Analytics
- Important Call Center Metrics and KPIs == Important Call Center Metrics and KPIs
- Important Call Center Analytic Platform Features == Important Call Center Analytic Platform Features
- Use the Best Call Center Analytics Software == Use the Best Call Center Analytics Software
What is Call Center Analytics?
Instead of call center leaders collecting, measuring, organizing, and reporting on performance metrics, call center analytics automates these processes to improve efficiencies, customer service, and ultimately the organization. The analytics aims to identify areas where improvements can be made–both within the call center and company-wide–and track the effects of those changes.
These platforms measure a wide range of metrics, including:
- Agent productivity
- Average call time
- Call abandonment rate
- Customer satisfaction
- Revenue
- Customer retention
- Customer effort score
- Service-level agreement (SLA) performance.
Call Center Analytics Benefits
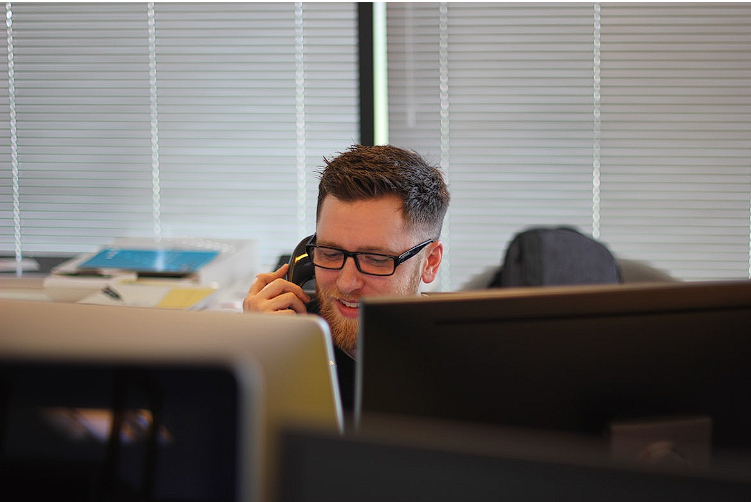
1. Stronger Agent Performance
Call center analytics tools track agent performance to uncover areas of excellence as well as opportunities for improvement. Call center leaders and coaches can objectively determine top performers via preset key performance indicators (KPIs), such as the hold time or first-call resolution for support agents and successful close rates or total deal value for sales reps.
Advanced analytics makes it possible to track individual performance across multiple metrics, giving agents the real-time feedback they need to build their skills and be successful. For example, Operative Intelligence’s analytics platform provides call centers with engaging, real-time dashboards that make it simple to identify areas for improvement based on customer needs. Users can see where performance variability is at a team and agent level so leaders can coach with precision and drive measurable improvements.
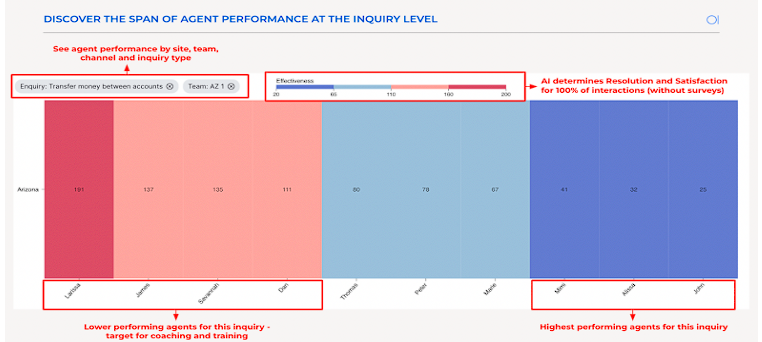
2. Improved Customer Satisfaction
To keep customers happy with a product or service, organizations need to be able to quickly identify customer pain points and areas of improvement. By leveraging call center analytics technology, call centers can track performance, see how different campaigns are performing, and understand where resources need to be allocated.
With these insights, call centers can respond quickly to customer inquiries, provide personalized service, create better strategies for improving customer retention and loyalty, and improve customer service metrics, like:
- First Response Time
- Call Handle Time
- First Call Resolution (FCR)
- Quality of Recent Support
- Average Wait Time
Call centers can even evaluate trends and anticipate customer needs before they even arise, ultimately leading to improved customer satisfaction over time.
3. Optimized Call Center Operations
By analyzing data points such as call duration, response time, and wait times, businesses can identify strengths and weaknesses within their operations. This process can lead to more efficient staffing and scheduling, better customer service strategies, and increased call completion rates.
Additionally, data analysis can uncover insights about customer preferences and behaviors that can be used to develop more effective marketing campaigns. With the right analytics tools, businesses have the potential to improve efficiency and profitability across all aspects of their call center operations.
The Operative Intelligence customer intelligence platform analyzes 100% of inbound interactions and shares the root causes of overall inquiries using the customer’s actual words. Organizations no longer struggle with finding and justifying which interactions could be automated, constructing a business case, and monitoring ROI.
With Operative Intelligence, this process is entirely automated–saving you months of time and effort–and the results are immediately available with a single click. You can be confident that the changes you’re making will give you the expected return through unique value scores for every driver.
Types of Call Center Analytics
The following are some of the common types of call center analytics.
Speech Analysis
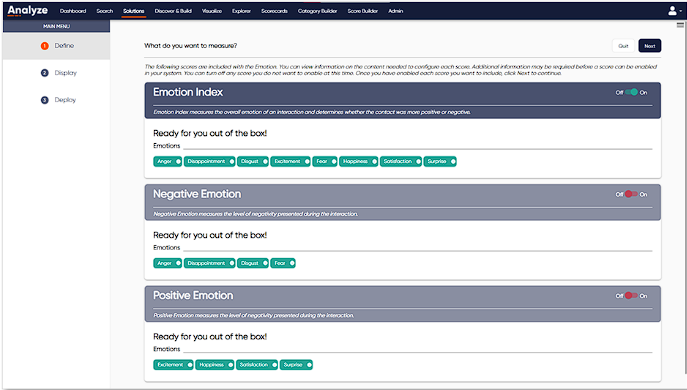
What it is:
Speech analytics utilizes advanced AI technology to comprehend, interpret, and analyze human speech. Speech analytics is becoming increasingly popular within call centers due to its ability to recognize speech accurately, convert it into text, and carry out sentiment analysis with remarkable precision.
Speech analytic software can analyze 100% of customer conversations, enabling call centers to understand precisely what transpires during the interactions. This allows call centers to be more proactive when dealing with customers.
Why it matters:
Speech analytics provides businesses with critical data that helps them understand their customers. By analyzing customers’ words, businesses can gain valuable insights into their customers' behavior and needs, enabling them to improve their products, services, and marketing strategies. This technology can also provide real-time feedback on customer service interactions and guide employees in providing better support.
Text Analysis
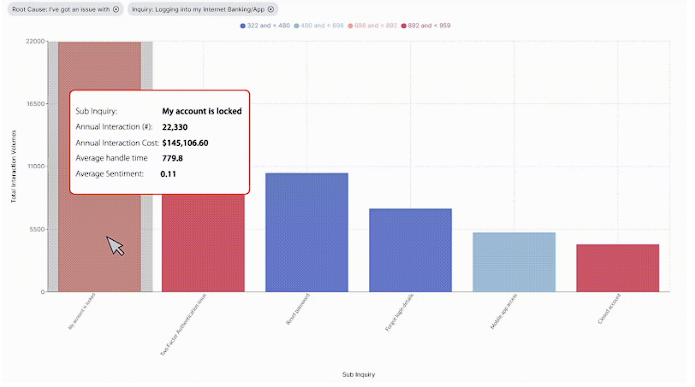
What it is:
Text analysis utilizes AI to uncover keywords and patterns in conversations between customers. Rather than focusing on verbal exchanges, they concentrate on written text such as documents, surveys, feedback forms, SMS messages, emails, and even social media posts.
Text analysis includes sentiment analysis and leverages machine learning and AI to discover the emotions behind the text. This helps organizations understand when and why customers are having either a positive or negative experience.
Why it matters:
Text analysis pulls data from customers’ own words to determine customer satisfaction levels, identify new markets and product opportunities, understand customers' needs and wants, and uncover macro trends in the customer experience. Thanks to this analysis, organizations can make better-informed decisions about their strategies and operations.
Interaction Analytics
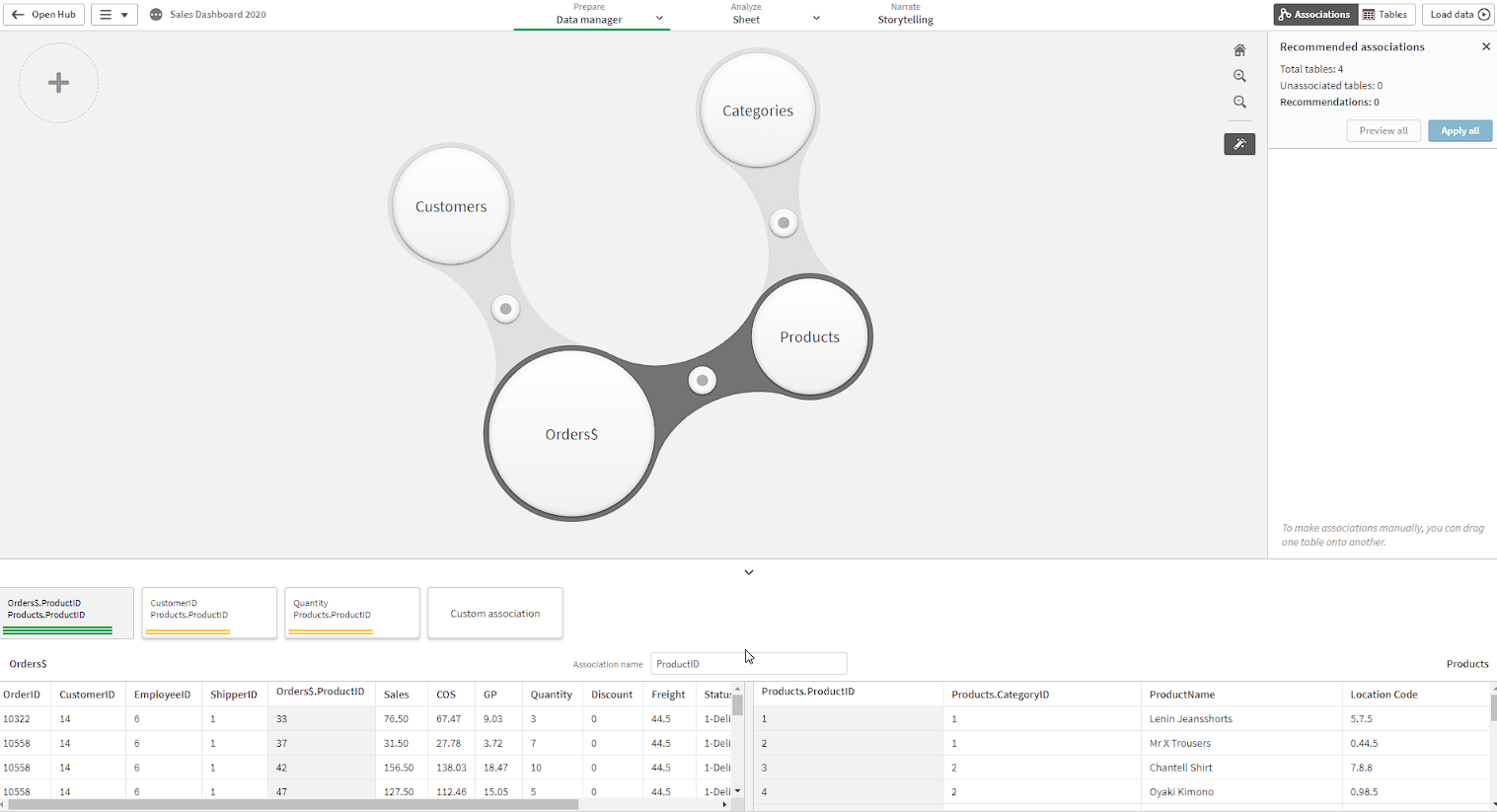
What it is:
Interaction analytics pulls customer data from multi-channel customer interactions and turns it into organized, easy-to-read reports. This form of analytics uses text and speech analysis, sentiment analysis, and predictive analytics to provide insights.
Why it matters:
Interaction analytics can help call centers gain valuable insight into customer behavior and interactions with their brand across various channels. This solution makes it easy for companies to measure multi-channel interactions to explore different aspects of the customer experience.
It also enables call centers to proactively address customer issues, manage quality assurance, and support agent training.
Self-service Analytics
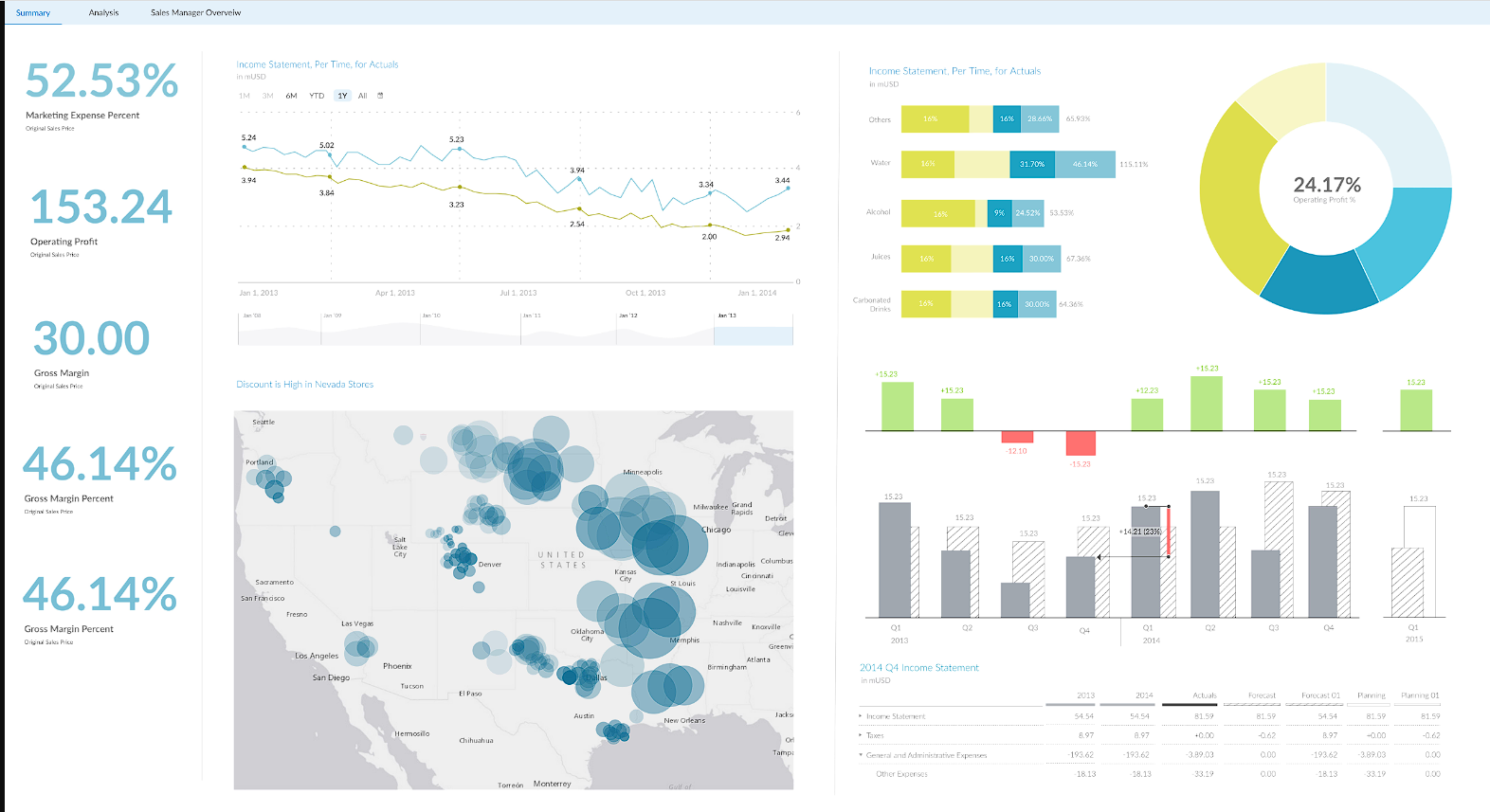
What it is:
Self-service analytics provides invaluable data from customer self-help portals such as FAQs, guides, and chatbots. By giving customers the capacity to solve their problems quickly, this support method can save time for both call center agents and customers.
Self-service analytics allows you to uncover the most common questions, phrases, and keywords used in searches on your website. For instance, if you discover that a frequent query is, “How long does shipping take?” then adding this information to your FAQ page can help reduce incoming calls for these matters all while optimizing the customer service experience.
Why it matters:
Self-service analytics makes call centers more efficient and cost-effective by reducing call times and providing solutions quickly. Call center leaders can identify customer needs, understand how customers interact with the website, gauge customer satisfaction, and develop better self-service options.
Call and contact centers can use self-service analytics to program chatbots and IVR features, and measure the success of a call center by tracking the call-to-action rate.
Predictive Analytics
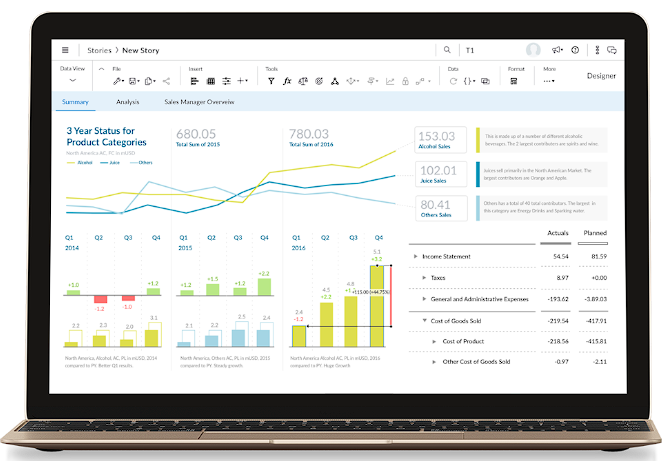
What it is:
Predictive analytics is an advanced call center analytics tool used to analyze past data to forecast future outcomes. Predictive analytics predicts call volume, call durations, call trends, customer satisfaction levels, and agent performance metrics. It also allows call center leaders to identify incoming call patterns and detect customer churn early.
Why it matters:
By leveraging predictive analytics, call center leaders can identify potential issues, make quick decisions, and offer precise solutions. Predictive analytics eliminates the guesswork by presenting data-driven predictions on what customers may do next. This maximizes workforce management, agent coaching, and call center efficiency.
Cross-channel Analytics
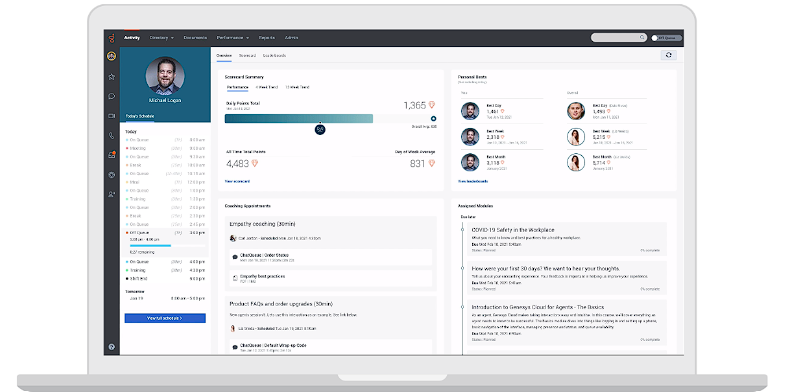
What it is:
Cross-channel analytics tracks customer interactions across various communication channels. This includes call centers, email, social media, chatbots, and more.
Why it matters:
Cross-channel analytics helps call centers to identify customer needs and preferences across all communication channels, as well as what customers’ preferred channels are.
By tracking customer journeys through different contact channels, call centers can also get an overall view of how customers are interacting with call centers and use this data to develop more effective call center strategies. It also helps customer experience teams create a more unified customer service experience.
Important Call Center Metrics and KPIs
The following are important metrics and KPIs that call centers can track to measure their performance.
After Call Work (ACW)
After-call work (ACW) is the period an agent spends after ending a call or other interaction that prevents them from starting any new tasks. During this interval, agents write notes, complete follow-up steps, or take short breaks.
Average Abandonment Rate (AAR)
Average Abandonment Rate (AAR) measures how many calls are abandoned in a queue before they’re answered. Call centers with long wait times also have high abandonment rates, representing a classic mismatch between supply and demand. Additional metrics can also include Calls Offered and Calls Answered, which are used to determine the abandonment rate.
Average Handle Time (AHT)
Average Handle Time (AHT) measures the amount of time frontline employees spend on servicing interactions, This can be tracked at an agent, team, or center level. Lower AHT not only reduces costs associated with service but assures customers that inquiries are being dealt with efficiently and effectively when associated with high rates of First Contact Resolution.
To keep their metrics healthy and consistent across agents, most call centers strive for a lower AHT rate as well as less variability between individual agents.
Average Hold Time (AHT)
Average Hold Time measures the amount of time that customers are being put on hold. It’s measured at the agent, team, and center levels.
Average Speed of Answer (ASA)
Average Speed of Answer (ASA) is a well-known benchmark in the customer service industry, indicating how long it usually takes for customers to have their calls answered. An industry standard that organizations often aim for is 80/20: eighty percent of calls are answered within twenty seconds or less.
Average Talk Time (ATT)
Average Talk Time (ATT) is a measurement of how long an agent and customer are conversing on the phone or, more specifically, the time when the customer is not on hold or the call has ended and the agent is completing other tasks.
For example, if AHT (Average Handling Time) was 540 seconds but ATT was 300 seconds, this means 240 seconds were used for other activities outside of talking with the caller.
Cost Per Call
The cost-per-call metric determines how much it costs a call center or company to handle a single call.
Customer Effort Score (CES)
The Customer Effort Score (CES) is an indicator of how much effort customers put in to complete their service requests. The higher the perceived effort, the lower customer satisfaction.
Customer Satisfaction (CSAT)
Customer Satisfaction (CSAT) is a metric used to measure how content customers are with their service experience. It's usually collected via an end-of-interaction survey, which can ask questions related to different aspects of the interaction.
First Call Resolution (FCR)
First Call Resolution (FCR) is the rate at which customers are having their inquiries resolved in one interaction. Traditionally, this was done through manual quality assessments or surveys - both of which have limitations on scalability and accuracy.
Now with Operative Intelligence, FCR can be accurately measured across large numbers of contacts without needing any kind of survey. FCR is recognized as an industry-leading measure and Operative Intelligence data has consistently shown that the higher the FCR, the higher the customer satisfaction.
Net Promoter Score (NPS)
Net Promoter Score (NPS) is another way to measure the customer’s experience with their interaction. It’s typically a macro measure of satisfaction since customers must answer how likely it is that they’d recommend a friend or colleague to an organization. This will often take into account the totality of a customer’s experience with the organization–not just the call center.
Repeat Calls
Repeat calls measure the amount of volume that is driven due to follow-ups (repeat calls). Repeat calls often occur because customers didn’t have their inquiry resolved the first time, so the customer needs to call them again for resolution.
Organizations will look at the phone numbers calling over a specific period to identify repeat traffic, but this doesn’t show them the reason behind the repeat contacts. Operative Intelligence can identify this data based on the language used by the customer when they contact, so organizations can resolve the core issue that’s driving customer calls.
Sentiment
Tracking sentiment is another way to measure a customer’s experience with their call center interaction. Sentiment analysis uses machine learning and AI to pull subjective elements from text to determine the feelings and opinions of the speaker. This can help organizations understand where and when customers are having a negative experience while using their product or service.
Service Level
Service-level metrics are a combination of metrics used throughout the industry and can often appear on KPIs along with CSAT or NPS scores. It often includes the speed of agent responses and abandonment rates.
Important Call Center Analytic Platform Features
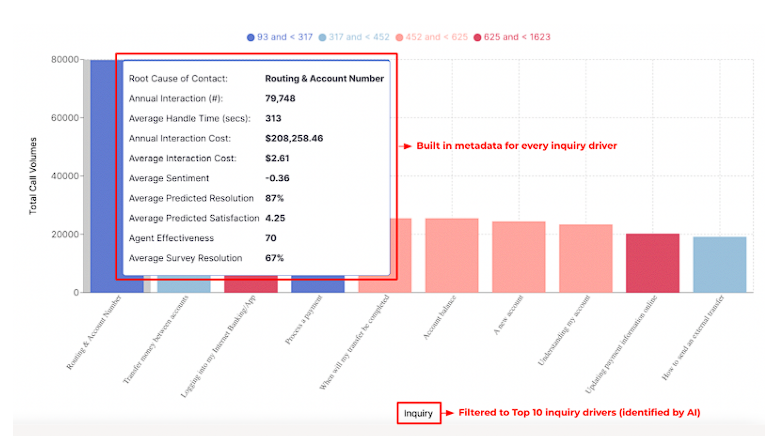
1. Actionable Insights
The data captured by an analytics platform should be meaningful and enable call center leaders to make tangible changes that impact customer satisfaction and call center performance.
Without real-world implications, data is just data. Call center leaders must be able to understand what actions to take based on the information provided.
2. Dashboards & Reports
Dashboards and reports empower managers to monitor their call center's operations, pinpoint areas that require improvement, and make decisions based on data-driven insights. It also reveals how distinct campaigns are performing and highlights the locations where resources must be assigned for maximum efficiency.
3. Clear ROI
An analytics platform should allow call centers to benchmark their performance against industry standards, identify key areas of improvement, and track the success of their initiatives over time.
Operative Intelligence analyzes 100% of inbound interactions and shares the root causes of overall inquiries using the customer’s actual words. Organizations no longer struggle with finding and justifying which interactions could be automated, constructing a business case, and monitoring ROI.
With Operative Intelligence, this process is entirely automated – saving you months of time and effort – and the results are immediately available with a single click. You can be confident that the changes you’re making will give you the expected return through unique value scores for every driver.
Use the Best Call Center Analytics Software
Advanced call center analytics helps customer experience teams put the customer first. But to fully realize the power of these analyses, call centers must use the right analytics software.
Operative Intelligence is a next-gen analytics platform for enterprise call and contact centers. Unlike traditional call center analytics tools, Operative Intelligence automatically transforms an organization's existing call center data into real-world, actionable insights. It pulls macro and micro cost insights that reveal:
- Customer pain points and what they cost the business
- What causes increases in handle time and cost and how to reduce it
- Opportunities for engineering teams to improve the product
- The return on investment (ROI) of potential changes
Ready to drive changes at every level of your business with irrefutable customer demand data?
Book a demo with Operative Intelligence today.